Building a career in emerging technologies is perhaps typical of a Cybernetic researcher. Kathy Reid, however, chose to study a foreign language alongside technology in her undergraduate degree, perhaps an influence from her childhood growing up around the famed Geordie accent. It is only fitting that Kathy’s PhD thesis in Cybernetics would be uniquely flavoured, merging her deep technological expertise with her life-long fascination with accents.
Here Kathy tells us how Cybernetics has guided her as a researcher and where to next once she’s completed her PhD.
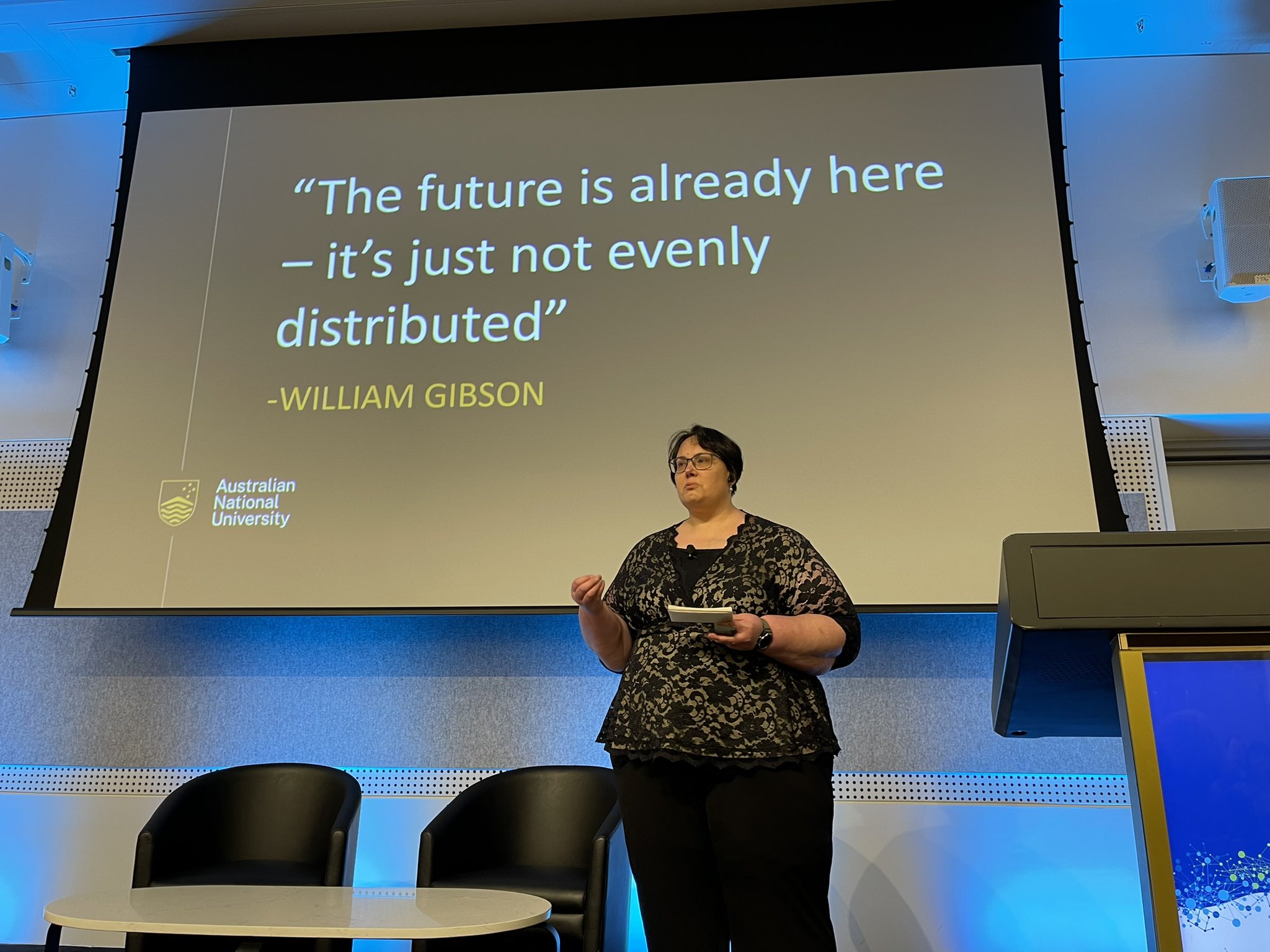
Tell us about your research and what led to your chosen thesis topic?#
I’ve been interested in accents from a young age and through circuitous means, I had an opportunity to work for a voice assistant company. The role excited me – voice-enabled technologies give people the ability to interact with the world through their speech – but I was struck by whose voices the technology would work for and whose voices it wouldn’t work for.
As a woman, the voice assistant often wouldn’t wake for me as the data had been trained by men with a deeper voice and a specific accent. The ability of the voice assistant to recognise accented speech was also impacted by the way the data had been trained.
There are more than 7000 languages spoken today, and by 2026 there are likely to be around 8.4 billion voice assistants in use globally, but these voice assistants are not built for everyone. I wanted to understand why speech recognition and voice assistant technologies work for some communities while not recognising the voices of others.
A lower literacy rate in some communities for example may lead to a greater reliance on speech recognition to search the internet, connect with family and friends, or access critical services like healthcare and banking. But if their voices aren’t recognised then access to these services is limited. Work still needs to be done to achieve inclusion and representation in voice-enabled technologies.
I’ve approached my research from all sorts of angles and I’ve landed on focusing on the role of voice data set documentation. This process includes components like data cards, model cards, how speech data and voice data is documented and what impact the documentation process has on the models that are trained. I also researched the way practitioners use that data through a set of interviews.
I should have stopped my research there and written it up and walked away with my PhD but then I became increasingly fascinated with accents and how they are represented.
I have been fortunate to work on incredible projects such as Mozilla’s Common Voice and during my time working on my PhD, OpenAI’s automatic speech recognition software called Whisper launched. It was evaluated for how accurate it was in understanding languages, but it wasn’t evaluated for how well it performed on particular accents. So, I tested Whisper using Mozilla’s Common Voice English dataset, which I had tagged for accents in a previous piece of research.
I found Whisper didn’t perform well on Scottish-accented English and many Asian accents of English. From this, I speculated Whisper didn’t have a lot of Scottish or Asian-accented English in its training set, but I can’t be sure of this because OpenAI is very guarded about where Whisper’s training data came from.
Ultimately, what I have found is, we need better standards of documenting speech data.
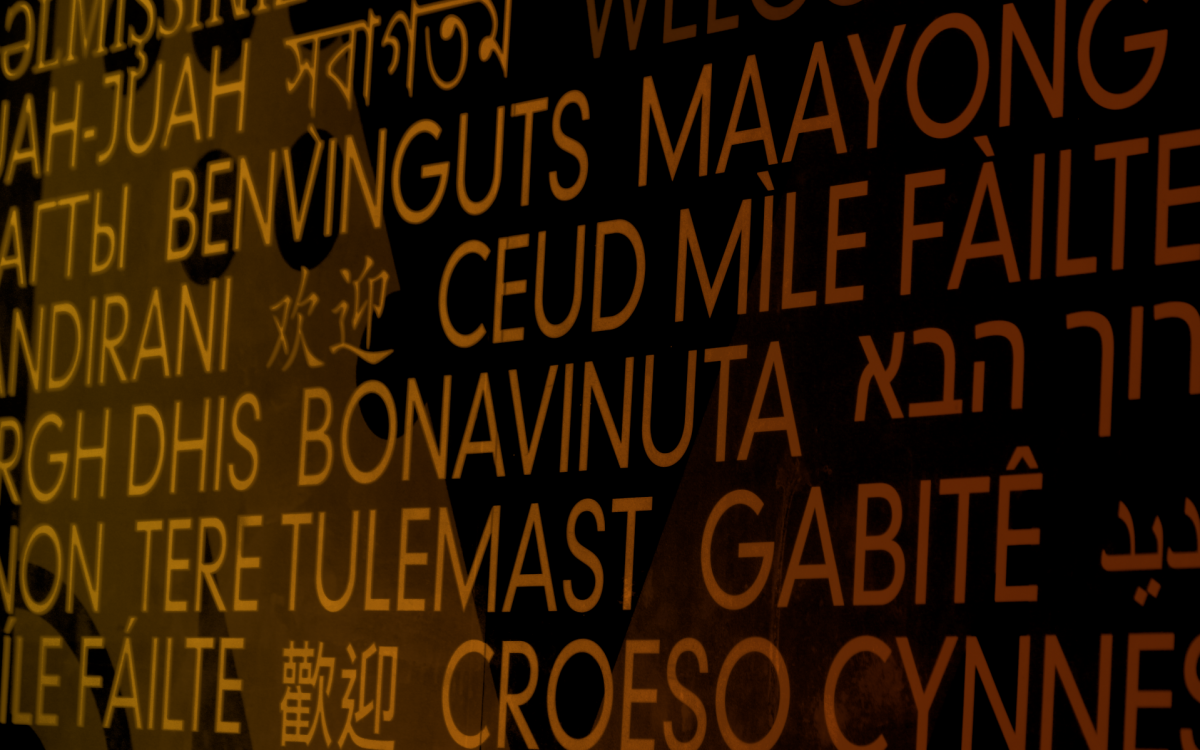
How has the school’s approach to Cybernetics, systems and futures shaped or guided you as a researcher?#
The systems focus of Cybernetics has been incredibly helpful at picking apart a lot of the thinking around data set documentation and asking what the underpinning is, or causal factor for, why we have speech technologies that don’t work well for all voices. In a traditional computer science area, it would more likely be a linear approach of A causes B, B causes C mindset.
But Cybernetics makes us think about the historical antecedents of the technologies we’re working with. If we look at the building blocks of speech for example, called phonemes, we can trace them back to the 4th century BC. In the 1960s, systems relied on recognising individual phonemes, then using rulesets would match those phonemes to words. The rulesets made the process more or less deterministic; if you had these phonemes, you had this word.
As computing power increased, there was a transition to statistical models that used probabilities to predict words from phonemes. While this allowed for prediction over much larger vocabularies, it also meant that speech recognition became probabalistic. The result was there was a chance it could get the transcription wrong, and today that is still the case.
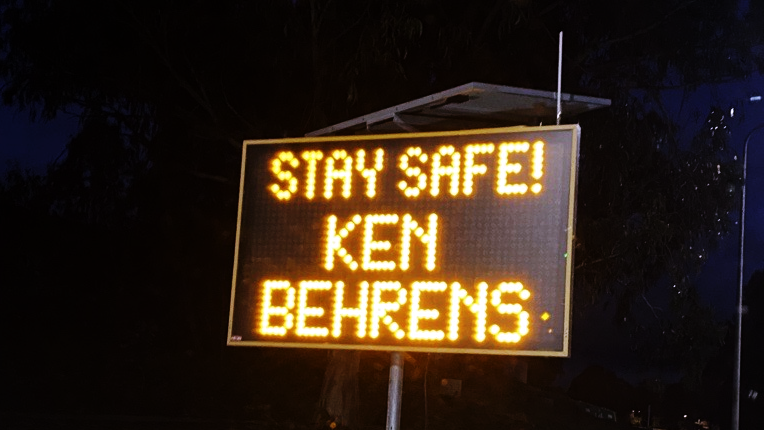
Ken Behrens is a case in point! Ken Behrens is a wildlife photographer in Madagascar but the reason Ken Behrens was predicted by the live transcription software during ACT Chief Minister’s press conference is there are more mentions of Ken Behrens in the training data than there are of the word Canberrans. The history of speech recognition helps us understand why some of these errors are happening today.
Cybernetics tells us that technologies always have a history, or multiple histories told by different people. The creator of a technology tells a different story to the user of a technology for example. Cybernetics also teaches us the future is never a given and if we want to live in a particular world, it’s beholden upon us to take actions that bring that world into being. It’s a proactive, action-focused discipline. That approach has been very important to my work as well.
How does your work relate to ANU’s specific and distinct mission to conduct research that transforms society and creates national capability?#
According to the 2021 census, about 250 or 300 languages are spoken in Australia. We’re losing Indigenous languages, but it’s not just language we’re losing, it is the knowledge and the culture and the heritage of the peoples who spoke that language. We’re currently in the decade of Indigenous languages, as deemed by UNESCO, and my work links to the ANU’s mission by recognising that Australia is not a monolingual country. We have incredible diversity in the languages spoken and when we think about AI and machine learning, what we’re seeing is a recolonisation of Australia.
The British colonisation of Australia resulted in the annihilation of Indigenous language, culture, and the denigration of a population. What we’re seeing now is techno colonisation. We have OpenAI, and Anthropic, and other competitors who are not Australian companies bringing their technology into Australia, and it’s not necessarily working in an Australian context.
My work contributes in a small way so that when we’re looking at AI and machine learning we are paying close attention to the diversity of the data that is being used, and the diversity of the people speaking to technology. We need to think about who’s speaking with machines, who the machine understands, and why.
How has this PhD shaped you on a personal level?#
It’s given me a much stronger appreciation for data as a cultural resource. My training and background as a technologist was focused on data as an asset, and something that is clean and ordered in a database.
Riffing off Geoffrey Bowker and Lisa Gitelman – data is never raw, it’s always cooked. Cybernetics has a strong anthropological and social science focus that teaches us to dig into how data is cooked, why it is cooked, and who’s doing the cooking.
I’ve always had a leaning towards technology as public good. And being at the School of Cybernetics has reinforced that, particularly from the perspective of having a moral obligation to create a better world, better than the one we found. That’s been a very strong throughline in all of the Cybernetic teachings.
Now we have been given the privilege of an incredible education and gained valuable skills, we have a responsibility to use them in a positive way for the world. My time at the School has reinforced that desire to do good with the skills that I have.
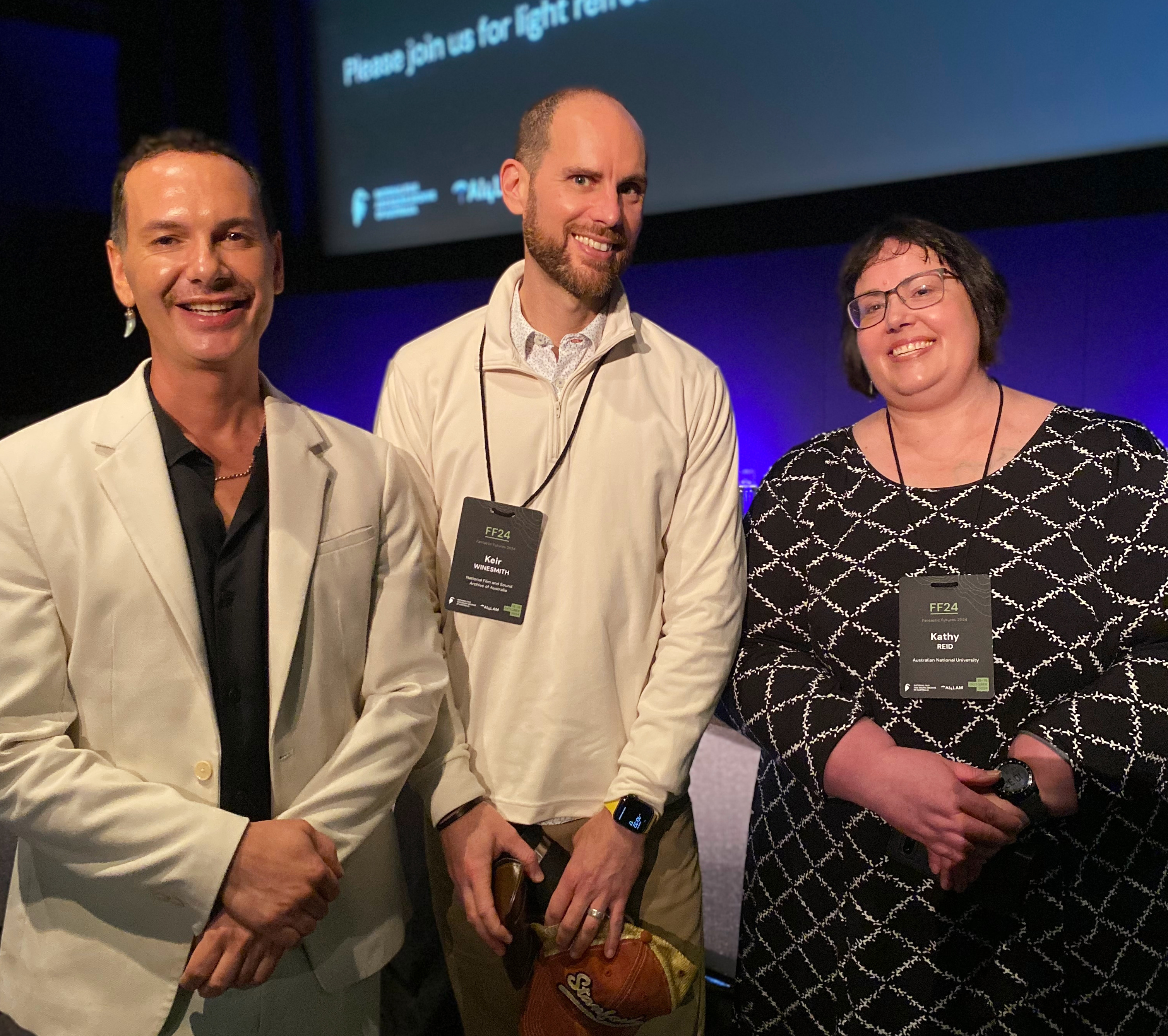
What’s next for you? How will you apply your talents and skills?#
I’ve had a varied career in emerging technology leading and managing projects and teams. I’ve worked in NVIDIA’s speech recognition team, one of the most technically advanced companies in the world, and I’ve worked at Mozilla in their Common Voice team on speech data – where I’m currently contracting. I’ve also worked with high-performance computing, where we use special hardware called GPUs, primarily for training machine learning models. But working on great projects or cutting-edge technology isn’t enough for me.
I really want an opportunity to continue growing my technical capability but in a way that helps people. So that could entail spearheading various technology revitalisation or speech data capture projects, potentially evaluating how well speech models work for particular cohorts, or how well they don’t work for particular cohorts.
I’d like to have a role to play in highlighting the dangers of distinguishing speech in different contexts. So, for example, there was a paper, where a government department in Turkey was able to distinguish the spoken accents of immigrants to determine where those immigrants came from geographically. Using the technologies that we have today for that sort of work has incredibly heavy moral implications.
Imagine if you were on the front line of an organisation, such as Services Australia, and through voice recognition technology were able to detect the accent of the person you were speaking with on the phone. The system might be able to detect they spoke with a particular type of Australian accent and because accent also denotes level of education, assumptions could potentially be made depending on the scenario. There could be a risk for automated accent bias. The risk for automated accent bias and automating the harms of accent detection, is something I don’t think we’re paying enough attention to. I would see myself in a role that helps to ameliorate or prevent those effects.
Wherever I go I’ll be using my technical skills to improve speech technologies so they are inclusive and representative.
Kathy’s research:#
- Everything you say to an Alexa Speaker will be sent to Amazon starting today
- In Conversation: Language, Culture and the Machines (2024)
- Common Voice and accent choice: data contributors self-describe their spoken accents in diverse ways (2023)
- Right the docs: Characterising voice dataset documentation practices used in machine learning (2023)
- Mozilla Common Voice v21 Dataset Metadata Coverage (2021)